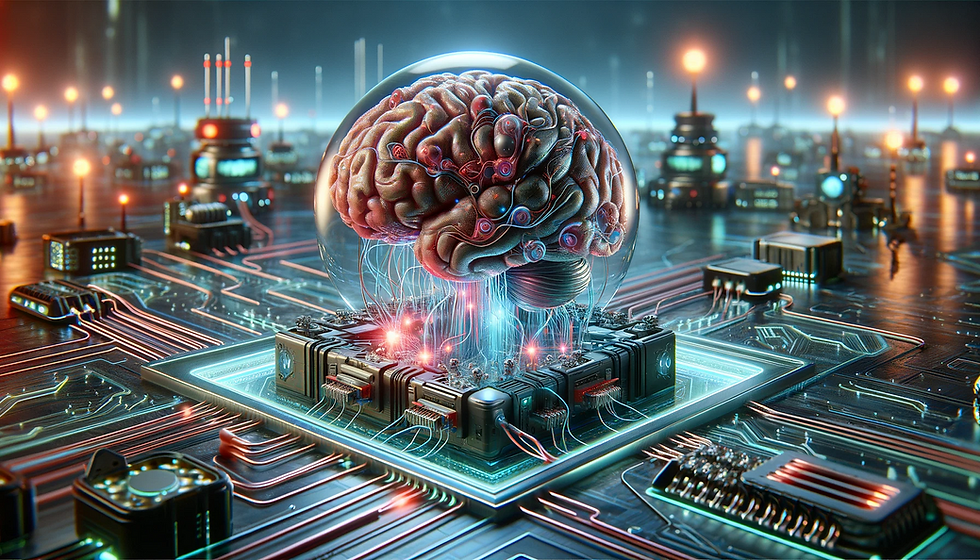
The 21st century has seen the rapid rise of Artificial Intelligence (AI), which is defined as “the ability of a computer or computer-controlled robot to perform tasks that are commonly associated with the intellectual processes characteristic of humans, such as the ability to reason”. It has become omnipresent by infiltrating all sectors of society, an example that may be familiar to most of us is Alexa- the AI modulated voice assistant introduced by Amazon. Whilst there is fear that the rapid development of the AI sector may lead to job deficiencies and insecurities, scientists are actively researching new methods to evolve our understanding of the application of artificial intelligence.
A breakthrough research study published by a team led by engineer Feng Guo of Indiana University Bloomington described in Nature on 11th December 2023, hopes to address current limitations in artificial intelligence technologies by simulating the neuroplasticity and structural functioning capabilities of the brain. In this approach, termed Brainoware, researchers built a hybrid computer using brain organoids (in-vitro stem cells that have the ability to mimic different types of cells, in this case the neurons of the brain) and electronic computer chips. In the past, scientists have seen great success in utilizing Artificial Neural Networks (ANNs) which are a computational mimicry of human neural networks that provide the computer an option to make human-like decisions. The ANNs have the ability to process large data sets via computer chips, however they provide for a difficult employment on current AI hardware as the process is highly energy exhaustive and time consuming. Hence, we see the emergence of Brainoware which aims to provide an energy efficient hardware solution to future attempts to produce neuromorphic chips with improved processing capabilities.
In the process of developing Brainoware, researchers connected a single organoid to a multitude of electrodes in order to connect live tissues to electronic circuits. The input information, i.e. the external stimuli, was transformed into electric pulses which were conveyed to the organoid system. The output, in the form of the organoid’s response to the input pulses, was detected by a sensor and fed into a machine-learning algorithm to decode it. There were multiple tests conducted to assess Brainoware’s processing capabilities. The physical capabilities of the system (eg. spatial processing information, fading memory etc.) were tested by analyzing response to multiple pulses and voltages. Next, it was tasked with solving real world problems such as speech recognition and nonlinear chaotic equation prediction (nonlinear equations are ones whose solutions are not analytical in nature, they are concerned with predicting phenomena such as chaos).
Brainoware showed a marked improvement from 51% to 78% in the speech recognition task over the testing period. Results indicated that the training led to changes in the organoid's functional connectivity in a way that resembles Unsupervised learning. Here Unsupervised learning refers to a type of machine-learning wherein a model learns patterns and relationships in data without explicit guidance or labeled examples. In the context of the organoid, changes in functional connectivity occurred without specific guidance, instructions or surveillance indicating a more autonomous adaptation or reorganization in response to the training. Thus, through training, Brainoware was able to improve upon its computational capabilities and display Unsupervised Learning abilities.
Despite all of its merits, Brainoware encounters many challenges including but not limited to organoid generation and maintenance and power consumption by external equipment. The organoid cells, unlike the computer chips, are living phenomena that must be grown arduously in incubators. The bigger problem is the task of keeping these living phenomena alive as they are highly susceptible to becoming necrotic (dead). I believe this is a great technological advancement that may bring fundamental changes to how we view biocomputing and AI as a whole. If further research leads to a potentially fruitful outcome, we can hope to see the application of this technique to improve speech recognition and nonlinear equation prediction in a reservoir computing framework.
Picture Credits: https://images.app.goo.gl/1s7THLsN8mwJ3Zeg6
Комментарии